Have you ever been denied a loan without fully understanding the reason? Or struggled to build credit due to a lack of traditional credit history? AI-based credit scoring is changing the game by providing more accurate, inclusive, and transparent financial assessments.
Unlike traditional methods that rely solely on factors like salary, debt, and repayment history, AI-driven models use a broader range of data. These models take into account factors like regular rent payments, freelance income, and even personal financial behaviors, which can be crucial for those outside the conventional credit systems. This approach opens up new opportunities for individuals who may have been overlooked by traditional credit scoring models.
However, that’s rapidly changing. Nearly 80% of organizations involved in credit risk are set to adopt generative AI within the next year, signifying a move towards smarter, quicker, and fairer systems.
This shift starts with the way we assess credit, and AI-based credit scoring models are already proving to be more insightful, adaptable, and efficient.
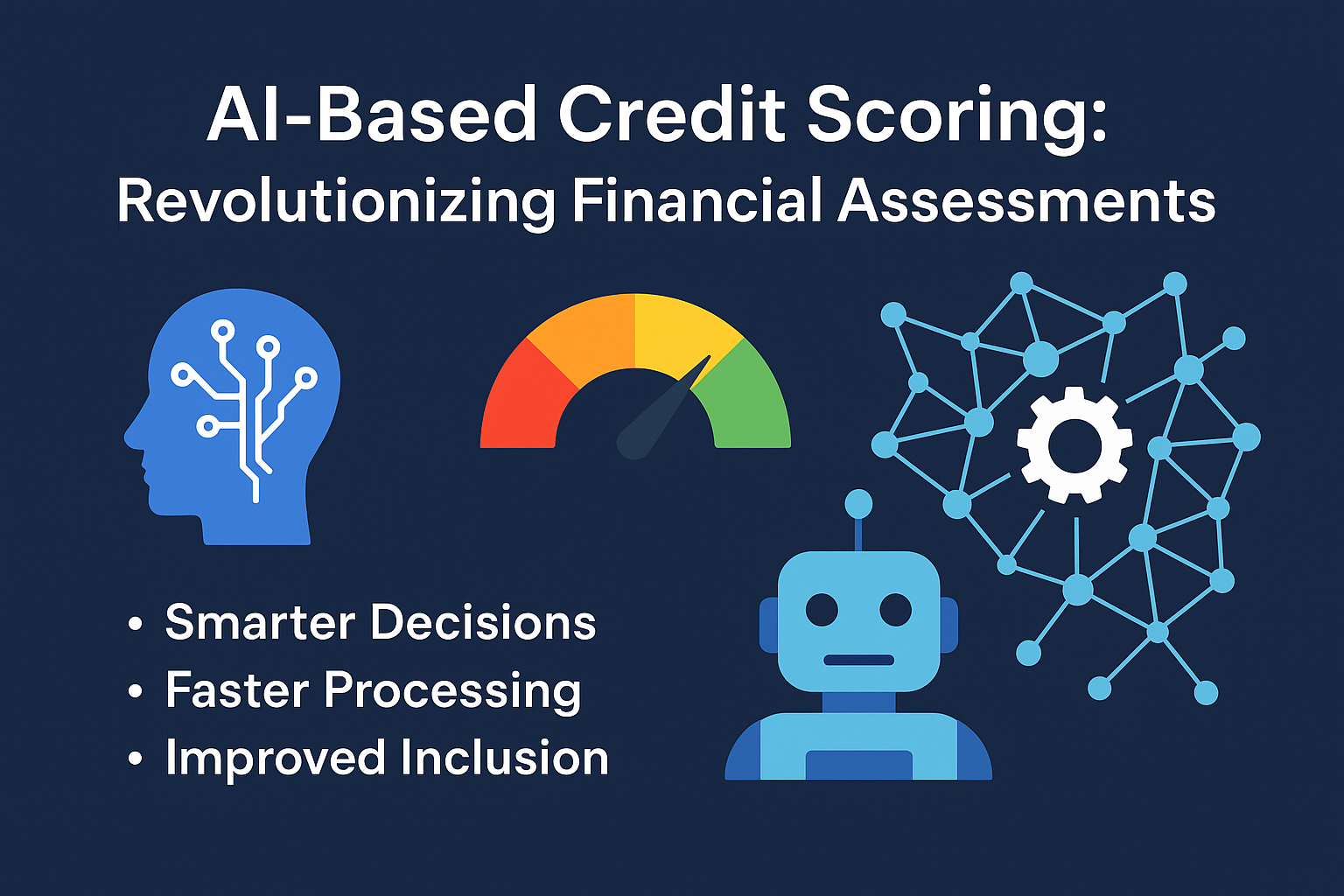
What is AI-Based Credit Scoring?
AI-based credit scoring uses artificial intelligence to evaluate an individual’s creditworthiness. Unlike traditional methods that depend solely on a credit report or income, AI models examine a broader set of data points and apply complex algorithms to assess lending risks more accurately.
This new approach is no longer just a theory—20% of financial institutions have already implemented at least one AI tool in their credit risk management. These tools range from early fraud detection to custom AI-powered credit scoring systems.
How It Works
Traditional credit models often fail to consider people who don’t have loans, mortgages, or long-term credit cards. This leads to challenges when evaluating younger individuals, gig workers, or residents of emerging economies.
AI credit scoring addresses these gaps by pulling data from a variety of sources, such as transaction histories, digital wallets, mobile phone usage, eCommerce receipts, and behavior on financial apps.
For example, consider a delivery driver paid regularly through a gig app. Even without a traditional credit card, AI can identify the stable income pattern and assess repayment potential.
AI is transforming credit evaluations, making them faster, more precise, and more inclusive. Many businesses are turning to advanced AI development services to create these next-gen scoring systems.
Key Differences Between Traditional and AI Credit Scoring
Traditional Model | AI Model |
---|---|
Based on credit history and income | Based on a mix of financial and behavioral data |
Updates slowly (monthly or longer) | Updates instantly with new data |
Rigid and limited to a few factors | Flexible, adjusting to user behavior |
Often excludes gig or informal workers | Includes individuals with non-traditional income |
With AI, applicants are evaluated based on current, actual behavior, not outdated templates. This is especially beneficial for individuals in regions where formal credit lines are rare.
How Does AI Improve Credit Scoring?
AI improves not only the scoring method but also the overall lending decision-making process—faster, broader, and with better outcomes for both lenders and borrowers.
For example, Okredo, a credit risk platform, raised funds to expand its AI-powered credit scoring system, specifically targeting small and medium-sized businesses (SMEs). Rather than focusing only on basic revenue figures, it analyzes supplier relationships, client turnover, and regional economic data, providing a much clearer financial picture than static balance sheets ever could.
Advantages
1. Accuracy
AI models can detect patterns that traditional models miss. For example, regular payments for rent or consistent phone data top-ups offer a much better insight into creditworthiness than a credit card payment from years ago. When tested against traditional models, AI systems often reduce false declines and flag potential defaulters early.
2. Inclusion
One major flaw of traditional credit models is the exclusion of individuals with little formal credit history—students, gig workers, or migrants, for example. In regions like Africa and Southeast Asia, fintech companies are already using AI-powered credit scoring to approve microloans based on alternative data sources like mobile usage or e-commerce transactions.
3. Efficiency
Traditional credit applications could take days or weeks to process, involving back-and-forth communication and paperwork. AI credit scoring speeds this up, processing vast amounts of data in seconds and making instant decisions on loan applications.
Criteria | Traditional Model | AI-Based Model |
---|---|---|
Application review | 2-7 days | Instant or within minutes |
Data points considered | 3-5 core indicators | Hundreds from structured/unstructured sources |
Adaptability | Quarterly updates | Real-time learning |
This rapid processing benefits both lenders and borrowers, reducing operational costs for lenders while enabling quicker loan approvals.
4. Adaptability
AI credit scoring models adapt to real-time changes in behavior. If a gig worker loses one source of income but gains another, their score will adjust. Similarly, if inflation affects spending patterns, the model will recalibrate to reflect these changes, ensuring that credit decisions are based on up-to-date data.
Challenges and Solutions
As AI becomes a more central tool in credit scoring, it brings new challenges. Key concerns include fairness, transparency, and data privacy.
1. Bias and Fairness
AI models can inherit biases from historical data. If past credit decisions were biased against certain demographics, an AI model trained on that data might perpetuate the same biases, leading to unfair results. This is why it’s essential to incorporate fairness filters during model training to avoid such issues.
2. Transparency
One of the biggest criticisms of AI-based credit scoring is that the decisions often feel like a “black box.” Users may be denied loans without understanding why, as AI models don’t always provide clear explanations for their decisions. This issue can be addressed through Explainable AI (XAI), which breaks down decisions into understandable steps.
3. Privacy
AI credit scoring requires the collection of a wide range of data, raising concerns about user privacy. It’s important that organizations comply with data privacy laws, such as the GDPR, and that users have control over what data is shared and how it’s used.
The Future of AI in Credit Scoring
AI-based credit scoring is not just a tool for the present; it’s the future. It offers greater accuracy, inclusion, and efficiency while addressing the challenges of traditional models. As financial institutions adopt AI, the technology is also evolving to ensure fairness, transparency, and privacy.
In the coming years, AI-powered credit scoring systems will continue to evolve, driven by new technologies such as blockchain, which could make credit data more secure and accessible across institutions globally. Additionally, AI’s role in emerging markets, where traditional banking is limited, will become increasingly significant, offering financial inclusion to underserved populations.
As more countries implement regulations governing AI in financial services, expect to see stricter standards on fairness, transparency, and data protection. These regulations will help refine AI credit scoring systems, ensuring they remain ethical and trustworthy.
Conclusion
AI is transforming the credit scoring landscape. By providing faster, more accurate, and inclusive credit assessments, AI is not only improving financial access for underserved populations but also reshaping the entire credit industry. As the technology continues to evolve, the next generation of AI credit scoring systems will be fairer, more transparent, and driven by user consent.